Thermal convection
"Turbulence" is often used as synonym for "complexity". It is a multi-scale phenomenon, showing chaotic behavior both in space and time. But it is also a phenomenon in which self-organization plays a central role. Weather, climate, and ocean flow are all prime examples of both turbulence and complexity. The advantage in turbulence is that the underlying dynamical equations are well-known. The most important way to drive turbulent flow is thermally - think of the atmosphere or of ocean flow. The paradigmatic example for such buoyancy driven flow is the Rayleigh-Bénard system, i.e., a fluid-filled cell heated from below and cooled from above. Next to the aspect ratio, defined by width of the cell divided by its height L, the central control parameters of the system are the Rayleigh number Ra (the dimensionless temperature difference between bottom and top plate) and the Prandtl number Pr (the ratio between kinematic viscosity and thermal diffusivity).
Heat transfer in a Rayleigh-Bénard system can satisfactory be described by the Grossmann-Lohse (GL) theory. In the GL-theory the Prandtl-Blasius boundary layer theory for laminar flow over an infinitely large plate was employed in order to calculate the thicknesses of the kinetic and thermal boundary layers, the kinetic and thermal dissipation rates, and finally these results were connected with the Rayleigh and Prandtl number dependence of the Nusselt number. Because the dynamics of the Rayleigh-Bénard system depends on the location, i.e. in the boundary layers or in the bulk, where most dissipation takes place, the Ra-Pr parameter space can be divided in different regimes as is shown in figure 1.
The following topics are specifically addressed:
High Rayleigh number thermal convection
Rotating Rayleigh-Bénard convection
2D Rayleigh-Bénard convection
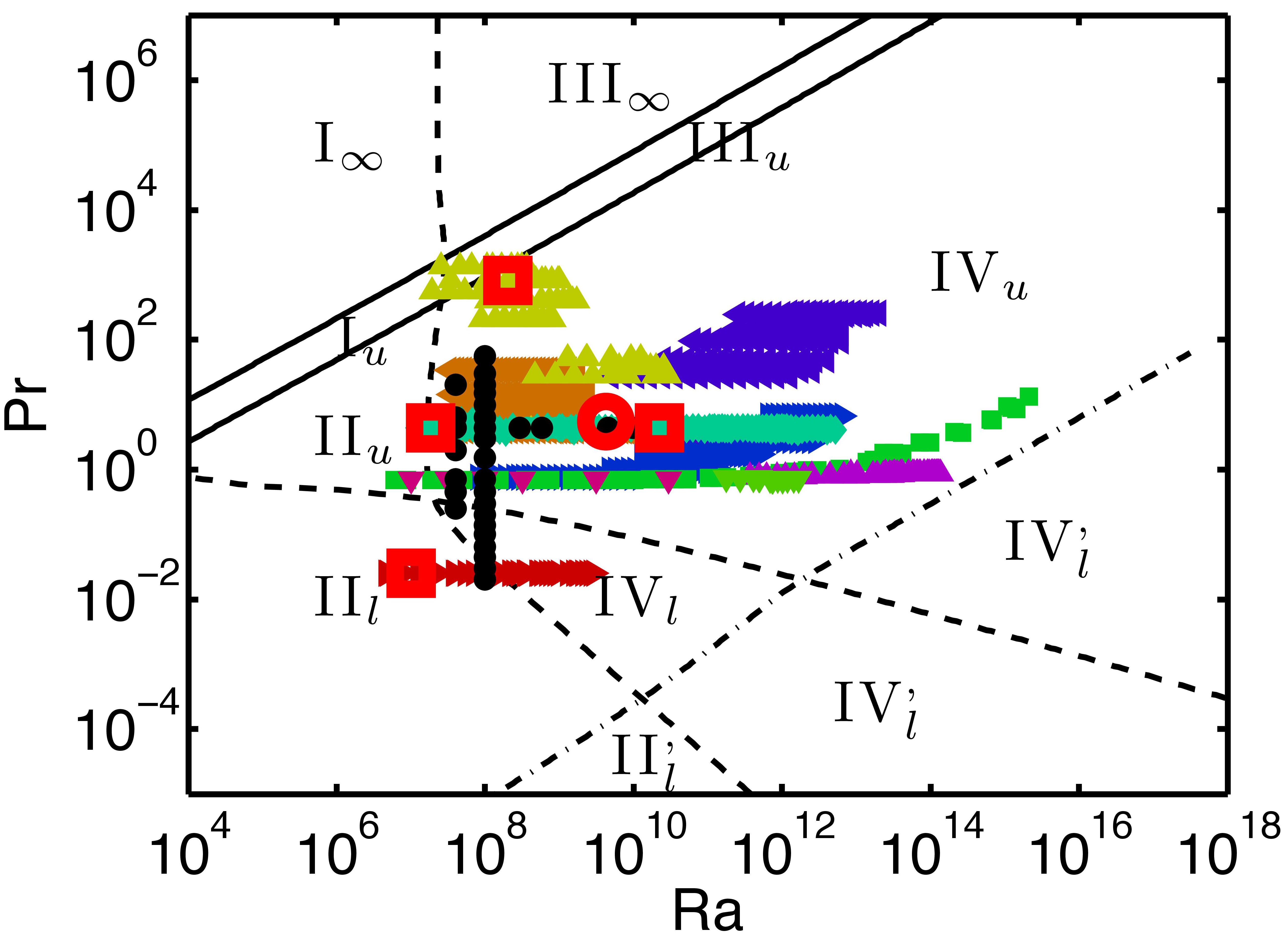
Figure 1: Phase diagram in Ra-Pr plane indicating the different turbulent regimes. The data points indicate where Nu has been measured or numerically calculated, figure from Ref. [3] where details about the shown data points can be found.
References
-
X. Zhu, E. Phillips, V. Spandan, J. Donners, G. Ruetsch, J. Romero, R. Ostilla-Mónico, Y. Yang, D. Lohse, R. Verzicco, M. Fatica, R.J.A.M. Stevens,
AFiD-GPU: a versatile Navier-Stokes Solver for Wall-Bounded Turbulent Flows on GPU Clusters,
Computer Physics Communications (2018) in press -
G.L. Kooij, M. A. Botchev, E.M.A. Frederix, B.J. Geurts, S. Horn, D. Lohse, E.P. van der Poel, O. Shishkina, R.J.A.M. Stevens, R. Verzicco,
Comparison of computational codes for direct numerical simulations of turbulent Rayleigh-Bénard convection,
Computer and Fluids 166, 1-8 (2018) -
X. Zhu, R.J.A.M. Stevens, R. Verzicco, D. Lohse,
Roughness-facilitated local 1/2 scaling does not imply the onset of the ultimate regime of thermal convection,
Submitted to Physical Review Letters (2017). -
J. Lülff, M. Wilczek, R.J.A.M. Stevens, R. Friedrich, D. Lohse,
Turbulent Rayleigh-Bénard convection described by projected dynamics in phase space,
J. Fluid Mech. 781, 276- 297 (2015).
-
R.J.A.M. Stevens, E.P. van der Poel, S. Grossmann, D. Lohse,
The unifying theory of scaling in thermal convection: The updated prefactors,
J. Fluid Mech. 730 , 295-308 (2013),
Second most cited J. Fluid Mech. article published since 2013.
-
R.J.A.M. Stevens, Q. Zhou, S. Grossmann, R. Verzicco, K.-Q Xia, D. Lohse,
Thermal boundary layer profiles in turbulent Rayleigh-Bénard convection in a cylindrical sample,
Phys. Rev. E 85, 027301 (2012).